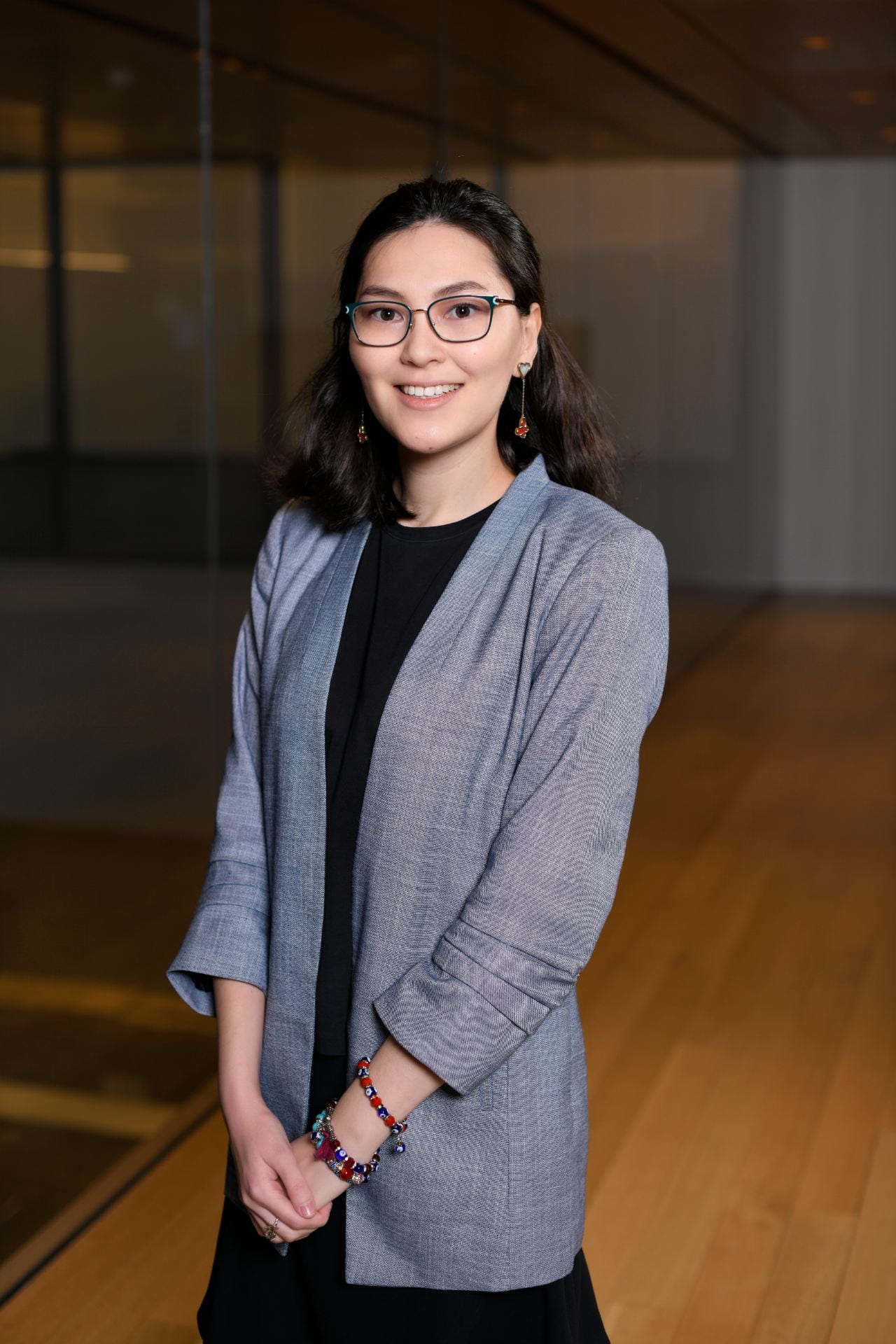
Malika Korganbekova
Quantitative Marketing
Contact Information
malika.korganbekova@kellogg.northwestern.edu
Faculty Advisors
Brett Gordon (Chair) Professor of Marketing
Eric Anderson (Co-Chair) Professor of Marketing
Robert Porter Professor of Economics
Caio Waisman Assistant Professor of Marketing
Research Interests
Digital Platforms, Privacy, Data Regulation, Sustainability
Methods: Field experimentation, Structural Econometrics, Deep Learning, Computer Vision and Multi-Armed Bandits
Job Market Paper
Balancing USER PRIVACY AND PERSONALIZATION (with Cole Zuber)
Awards: MSI A.G. Clayton Best Dissertation Proposal 2022 and Shankar-Spiegel Best Dissertation Proposal Award 2023
Privacy restrictions imposed by browsers such as Safari and Chrome limit the quality of individual-level data used in personalization algorithms. This paper investigates the consequences of these privacy restrictions on consumer, seller and platform outcomes using data from Wayfair, a large US-based online retailer. Large-scale randomized experiments indicate that personalization increases seller and platform revenue, and leads to better consumer-product matches with 10% lower post-purchase product returns and 2.3% higher repeat purchase probability. Privacy restrictions can distort these benefits because they limit platforms’ ability to personalize. We find that two main policies imposed by Safari and Chrome disproportionately hurt price responsive consumers and small/niche product sellers. To address this, we propose and evaluate a probabilistic recognition algorithm that associates devices with user accounts, even without exact user identity. Our findings demonstrate that this approach mitigates much of welfare and revenue losses, striking a balance between privacy and personalization.
Working Papers
Assessing the Environmental Impact of Fast Shipping: Towards Sustainable Ranking Solutions (with Aliya Korganbekova, Yasaman Khazaeni and Cole Zuber)
Presentations: INFORMS Conference, Rising Scholars Conference at UChicago, CODE at MIT, UT Austin Sheth Consortium, Transatlantic Doctoral Conference at LBS
Media Coverage: Invited for ORMS Today INFORMS Magazine submission
The environmental impact of online retailers, particularly in relation to fast delivery promises, is a growing concern for policymakers. Using experimental and observational data from an online retail platform, Wayfair, we investigate the causal impact of platforms’ shipping strategies on environmental emissions. We use a large-scale A/B test where Wayfair removed fast shipping badges to show that consumers who didn’t see fast shipping badges substituted to products from more distant warehouses. Contrary to policymakers’ expectations, removing fast shipping badges led to higher environmental emissions. Moreover, consumers paid higher shipping costs (+2.9%), bought more expensive items (+4.9%) and had longer wait times (+2.3%). We propose a product ranking algorithm designed to nudge consumers towards more environmentally friendly products. We demonstrate that our algorithm can lower emissions and shipping costs without compromising conversion and revenue metrics.
Regulating Data Usage on Dual Platforms (Sole AUthor)
Presentations: Marketing Science 2023, Platforms Symposium at Boston University 2023. Accepted for presentations at USC Artificial Intelligence & Management Conference, Digital Economics Paris and Theory and Practice in Marketing at the University of Lausanne
I examine the necessity and the design of data usage regulation in B2B markets, particularly focusing on concerns regarding platforms like Amazon that function as both marketplaces and sellers. The key issue is the alleged use of internal Amazon data to replicate top-performing products from third-party sellers. Using Deep Learning tools, I analyze visual and textual similarity measures between 624 Amazon Basics and 2 million third-party seller products. The findings reveal significant and consistent similarities between private label and third-party products across multiple product categories. Additionally, my research questions the effectiveness of duration-based regulation proposed by the European Union. It shows that Amazon takes an average of 2.5-3 years to imitate a product, while smaller sellers’ products require approximately 5 years. This indicates that duration-based data regulation provides Amazon with ample time to collect data, suggesting the need for a different seller-targeted data regulation approach.
Ranking algorithms and Equilibrium prices (With Yufeng Huang and Aliya Korganbekova)
Presentations: Marketing Science 2022 and Wharton Innovation Doctoral Symposium
We examine the impact of ranking algorithms on equilibrium prices and product quality in e-commerce platforms. Sellers may strategically set prices to affect future rankings, leading to differentiated pricing based on their private information about product quality. We collaborate with a major e-commerce platform and utilize experimental variations in algorithm components to analyze sellers’ equilibrium strategies. Through an experimental “boost” to product rankings, we observe that initial ranking improvements lead to increased traffic and sales. High-quality sellers respond by reducing wholesale prices by 2.2-3.5% to maintain their ranking advantage, while low-quality sellers raise wholesale prices by 1.5% for short-term profit gains. As a result, rankings impact equilibrium prices, creating sorting effects that unveil sellers’ quality and expedite quality disclosure. We develop a calibrated model to match the experimental findings and evaluate alternative policies wherein the platform boosts the products with the most quality uncertainty to accelerate quality revelation.
Work In Progress
Optimal targeting of exclusive products (with Alex Mackay)
Online retail platforms carry exclusive products to differentiate themselves from competitors. However, there could be adverse selection in products that opt-in to exclusivity. To address this concern, the online platform we collaborate with employs a combination of human judgment and algorithmic methods to determine which products should be offered exclusively. We exploit variation in the selection method and algorithmic scores to quantify the impact of exclusivity using Regression Discontinuity Design. Preliminary findings suggest that algorithm-chosen exclusive products get 10% higher revenue than similar non-exclusive products, while human-chosen exclusive products have 5% higher revenue. However, we also find that algorithm-chosen exclusive products tend to increase their prices after becoming exclusive. To investigate the underlying effectiveness of human versus algorithm-based product selection, we develop a dynamic model of exclusivity targeting. The model enables us to explore alternative approaches to selecting products for exclusivity to mitigate the adverse selection issue.
Probabilistic Identity Recognition for personalization (With Cole Zuber)
Privacy restrictions impact traditional cookie-based identity recognition practices. Therefore, online platforms face fragmented user data instead of having a single view of the consumers’ browsing journey across multiple devices and browsers. We propose a probabilistic identity recognition algorithm to help online platforms recognize consumers without using exact user identity. The algorithm takes as an input consumers’ browsing behavior and device characteristics to probabilistically predict the device’s association with the existing user account. We show that conditional on detailed behavioral data, XGBoost-based algorithm can achieve high levels of accuracy. The probabilistic solutions can help platforms continue personalizing user experience without compromising user privacy.